- Beranda
- Komunitas
- Story
- penelitian
Literature Review and Theoretical Review of Adaptive Learning Systems
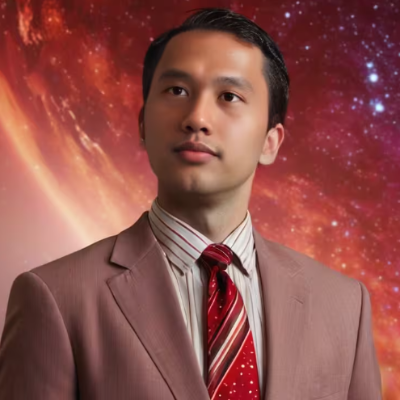
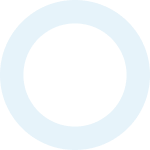
TS
yuliuseka
Literature Review and Theoretical Review of Adaptive Learning Systems
Literature Review and Theoretical Review of Adaptive Learning Systems
Introduction
Adaptive Learning Systems (ALS) are educational technologies that customize the learning experience for individual students based on their unique needs, preferences, and performance. These systems leverage various methodologies from artificial intelligence (AI), machine learning (ML), and cognitive science to create personalized learning paths that aim to optimize educational outcomes. This review explores the theoretical foundations, methodologies, applications, and challenges associated with Adaptive Learning Systems.
Literature Review
Historical Development
The concept of adaptive learning dates back to the 1970s with the advent of computer-assisted instruction (CAI). Early systems used simple algorithms to adjust the difficulty of questions based on student performance. With advancements in AI and ML, modern adaptive learning systems have become far more sophisticated, capable of analyzing vast amounts of data to provide highly personalized learning experiences.
Key Concepts and Techniques
[color=var(--tw-prose-bold)]Data Collection and Analysis:
Learner Profiling: Collecting data on students' learning styles, preferences, and prior knowledge to build a comprehensive learner profile.
Behavioral Data: Analyzing students' interactions with the system, such as time spent on tasks, types of errors made, and patterns of engagement.
Personalization Algorithms:
Rule-Based Systems: Using predefined rules to adapt content and assessments based on learner profiles and performance.
Machine Learning Models: Applying algorithms like decision trees, clustering, and collaborative filtering to predict student needs and recommend appropriate resources.
Reinforcement Learning: Employing reinforcement learning techniques to dynamically adjust learning paths based on ongoing student performance.
Content Adaptation:
Adaptive Content Delivery: Modifying the sequence, difficulty, and format of content delivery based on individual learner needs.
Intelligent Tutoring Systems (ITS): Providing real-time feedback and guidance to students through conversational agents or virtual tutors.
Assessment and Feedback:
Formative Assessment: Continuously assessing student performance to provide immediate feedback and adjust learning paths.
Summative Assessment: Evaluating overall learning outcomes to determine the effectiveness of the adaptive learning system.
[/color]
Applications
Adaptive Learning Systems have a wide range of applications across various educational and training contexts:
[color=var(--tw-prose-bold)]K-12 Education: Personalizing learning experiences to address diverse student needs and improve academic outcomes.
Higher Education: Enhancing learning engagement and retention by providing customized learning resources and support.
Corporate Training: Tailoring training programs to individual employee needs to improve skill development and job performance.
Special Education: Supporting students with disabilities by providing personalized learning interventions.
[/color]
Challenges
[color=var(--tw-prose-bold)]Data Privacy and Security: Ensuring the privacy and security of sensitive student data.
Scalability: Developing systems that can effectively scale to accommodate large numbers of students.
Interoperability: Ensuring that adaptive learning systems can integrate with other educational technologies and platforms.
Bias and Fairness: Addressing potential biases in data and algorithms to ensure fair and equitable learning experiences.
[/color]
Theoretical Review
Theoretical Foundations
Adaptive Learning Systems are grounded in various theoretical frameworks from education, cognitive science, and computer science:
[color=var(--tw-prose-bold)]Educational Theories:
Constructivism: Emphasizes the active role of learners in constructing knowledge through experience and reflection.
Zone of Proximal Development (ZPD): Suggests that learning is most effective when instruction is tailored to the learner's current developmental level.
Cognitive Theories:
Cognitive Load Theory: Focuses on optimizing the cognitive load during learning to enhance comprehension and retention.
Metacognition: Involves teaching learners to think about their own thinking processes to improve self-regulation and learning strategies.
Artificial Intelligence and Machine Learning:
Supervised Learning: Involves training models on labeled data to make predictions about student needs and performance.
Unsupervised Learning: Uses clustering and other techniques to identify patterns and group similar learners without labeled data.
Reinforcement Learning: Models the learning process as a series of decisions, using feedback to improve future actions.
[/color]
Evaluation Metrics
Common metrics used to evaluate Adaptive Learning Systems include:
[color=var(--tw-prose-bold)]Learning Gain: Measures the improvement in student knowledge or skills as a result of using the adaptive learning system.
Engagement Metrics: Tracks student interactions with the system, such as time on task, click-through rates, and completion rates.
Accuracy of Recommendations: Evaluates how well the system's recommendations align with student needs and preferences.
User Satisfaction: Assesses students' and educators' satisfaction with the adaptive learning experience.
[/color]
Future Directions
Future research in Adaptive Learning Systems may focus on:
[color=var(--tw-prose-bold)]Advanced Personalization Techniques: Leveraging deeper insights from AI and ML to further refine personalization.
Adaptive Assessments: Developing more sophisticated adaptive assessment tools that can provide real-time feedback and adjust learning paths accordingly.
Cross-Platform Integration: Ensuring seamless integration of adaptive learning systems with other educational tools and platforms.
Ethical Considerations: Addressing ethical issues related to data privacy, bias, and the transparency of AI algorithms.
[/color]
Conclusion
Adaptive Learning Systems have evolved significantly, from simple rule-based approaches to sophisticated AI-driven models. These systems offer the potential to revolutionize education by providing highly personalized learning experiences that cater to individual student needs. However, challenges such as data privacy, scalability, and fairness must be addressed to fully realize the benefits of adaptive learning. Continued research and innovation are essential to advancing the field and ensuring that adaptive learning systems can meet the diverse needs of learners in various educational contexts.
Introduction
Adaptive Learning Systems (ALS) are educational technologies that customize the learning experience for individual students based on their unique needs, preferences, and performance. These systems leverage various methodologies from artificial intelligence (AI), machine learning (ML), and cognitive science to create personalized learning paths that aim to optimize educational outcomes. This review explores the theoretical foundations, methodologies, applications, and challenges associated with Adaptive Learning Systems.
Literature Review
Historical Development
The concept of adaptive learning dates back to the 1970s with the advent of computer-assisted instruction (CAI). Early systems used simple algorithms to adjust the difficulty of questions based on student performance. With advancements in AI and ML, modern adaptive learning systems have become far more sophisticated, capable of analyzing vast amounts of data to provide highly personalized learning experiences.
Key Concepts and Techniques
[color=var(--tw-prose-bold)]Data Collection and Analysis:
Learner Profiling: Collecting data on students' learning styles, preferences, and prior knowledge to build a comprehensive learner profile.
Behavioral Data: Analyzing students' interactions with the system, such as time spent on tasks, types of errors made, and patterns of engagement.
Personalization Algorithms:
Rule-Based Systems: Using predefined rules to adapt content and assessments based on learner profiles and performance.
Machine Learning Models: Applying algorithms like decision trees, clustering, and collaborative filtering to predict student needs and recommend appropriate resources.
Reinforcement Learning: Employing reinforcement learning techniques to dynamically adjust learning paths based on ongoing student performance.
Content Adaptation:
Adaptive Content Delivery: Modifying the sequence, difficulty, and format of content delivery based on individual learner needs.
Intelligent Tutoring Systems (ITS): Providing real-time feedback and guidance to students through conversational agents or virtual tutors.
Assessment and Feedback:
Formative Assessment: Continuously assessing student performance to provide immediate feedback and adjust learning paths.
Summative Assessment: Evaluating overall learning outcomes to determine the effectiveness of the adaptive learning system.
[/color]
Applications
Adaptive Learning Systems have a wide range of applications across various educational and training contexts:
[color=var(--tw-prose-bold)]K-12 Education: Personalizing learning experiences to address diverse student needs and improve academic outcomes.
Higher Education: Enhancing learning engagement and retention by providing customized learning resources and support.
Corporate Training: Tailoring training programs to individual employee needs to improve skill development and job performance.
Special Education: Supporting students with disabilities by providing personalized learning interventions.
[/color]
Challenges
[color=var(--tw-prose-bold)]Data Privacy and Security: Ensuring the privacy and security of sensitive student data.
Scalability: Developing systems that can effectively scale to accommodate large numbers of students.
Interoperability: Ensuring that adaptive learning systems can integrate with other educational technologies and platforms.
Bias and Fairness: Addressing potential biases in data and algorithms to ensure fair and equitable learning experiences.
[/color]
Theoretical Review
Theoretical Foundations
Adaptive Learning Systems are grounded in various theoretical frameworks from education, cognitive science, and computer science:
[color=var(--tw-prose-bold)]Educational Theories:
Constructivism: Emphasizes the active role of learners in constructing knowledge through experience and reflection.
Zone of Proximal Development (ZPD): Suggests that learning is most effective when instruction is tailored to the learner's current developmental level.
Cognitive Theories:
Cognitive Load Theory: Focuses on optimizing the cognitive load during learning to enhance comprehension and retention.
Metacognition: Involves teaching learners to think about their own thinking processes to improve self-regulation and learning strategies.
Artificial Intelligence and Machine Learning:
Supervised Learning: Involves training models on labeled data to make predictions about student needs and performance.
Unsupervised Learning: Uses clustering and other techniques to identify patterns and group similar learners without labeled data.
Reinforcement Learning: Models the learning process as a series of decisions, using feedback to improve future actions.
[/color]
Evaluation Metrics
Common metrics used to evaluate Adaptive Learning Systems include:
[color=var(--tw-prose-bold)]Learning Gain: Measures the improvement in student knowledge or skills as a result of using the adaptive learning system.
Engagement Metrics: Tracks student interactions with the system, such as time on task, click-through rates, and completion rates.
Accuracy of Recommendations: Evaluates how well the system's recommendations align with student needs and preferences.
User Satisfaction: Assesses students' and educators' satisfaction with the adaptive learning experience.
[/color]
Future Directions
Future research in Adaptive Learning Systems may focus on:
[color=var(--tw-prose-bold)]Advanced Personalization Techniques: Leveraging deeper insights from AI and ML to further refine personalization.
Adaptive Assessments: Developing more sophisticated adaptive assessment tools that can provide real-time feedback and adjust learning paths accordingly.
Cross-Platform Integration: Ensuring seamless integration of adaptive learning systems with other educational tools and platforms.
Ethical Considerations: Addressing ethical issues related to data privacy, bias, and the transparency of AI algorithms.
[/color]
Conclusion
Adaptive Learning Systems have evolved significantly, from simple rule-based approaches to sophisticated AI-driven models. These systems offer the potential to revolutionize education by providing highly personalized learning experiences that cater to individual student needs. However, challenges such as data privacy, scalability, and fairness must be addressed to fully realize the benefits of adaptive learning. Continued research and innovation are essential to advancing the field and ensuring that adaptive learning systems can meet the diverse needs of learners in various educational contexts.
0
3
1
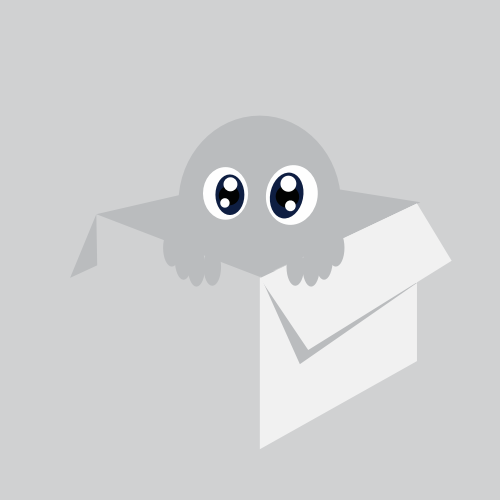
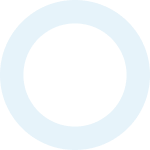
Komentar yang asik ya
Urutan
Terbaru
Terlama
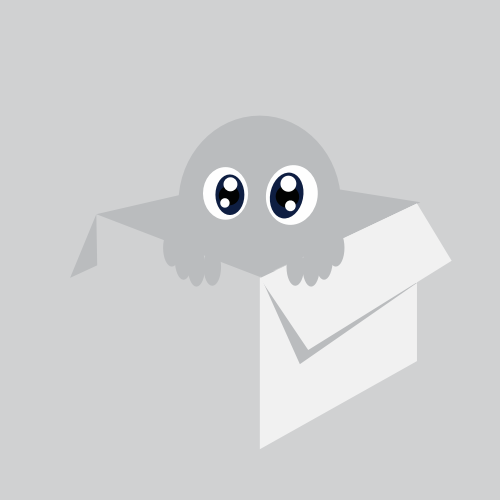
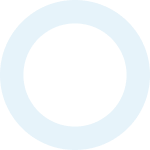
Komentar yang asik ya
Komunitas Pilihan