- Beranda
- Komunitas
- Story
- penelitian
Literature Review and Theoretical Review of Social Network Analysis (SNA)
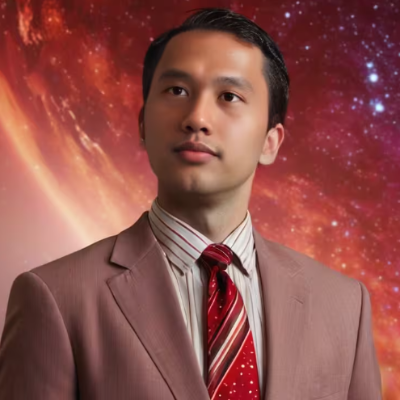
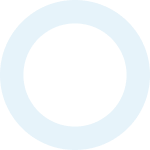
TS
yuliuseka
Literature Review and Theoretical Review of Social Network Analysis (SNA)
Literature Review and Theoretical Review of Social Network Analysis (SNA)
Introduction
Social Network Analysis (SNA) is a multidisciplinary field that examines social structures through the lens of networks. It explores the relationships and interactions among individuals, groups, organizations, or other social entities. This review provides an overview of the theoretical foundations, methodologies, applications, and challenges associated with Social Network Analysis.
Literature Review
Historical Development
The origins of Social Network Analysis can be traced back to the early 20th century, with the work of sociologists such as Georg Simmel and Jacob Moreno. However, it gained prominence in the latter half of the century, particularly with the development of mathematical graph theory and advancements in computing technology. Today, SNA is widely used across disciplines such as sociology, anthropology, psychology, economics, biology, and computer science.
Key Concepts and Techniques
[color=var(--tw-prose-bold)]Network Representation:
Social networks are typically represented as graphs, with nodes representing individual actors or entities and edges representing relationships or interactions between them. Various types of networks exist, including directed and undirected networks, weighted networks, and multiplex networks, each capturing different aspects of social structure.
Centrality Measures:
Centrality measures, such as degree centrality, betweenness centrality, and eigenvector centrality, quantify the importance or influence of nodes within a network. These measures help identify key actors or entities that play pivotal roles in information flow, collaboration, or influence propagation.
Community Detection:
Community detection algorithms identify densely connected groups or communities within a network. These algorithms partition the network into cohesive clusters based on the strength of connections between nodes, revealing underlying structures and patterns of organization.
Network Visualization:
Network visualization techniques enable the visual exploration and analysis of social networks. Graph visualization tools and software packages allow researchers to interactively visualize large-scale networks, identify patterns, and gain insights into network structure and dynamics.
Statistical Models:
Statistical models, such as exponential random graph models (ERGMs) and stochastic actor-oriented models (SAOMs), are used to infer generative processes underlying observed network data. These models provide a probabilistic framework for analyzing network evolution, formation, and dynamics.
[/color]
Applications of Social Network Analysis
Social Network Analysis has diverse applications across various domains, including sociology, organizational studies, epidemiology, information science, marketing, and cybersecurity. In sociology, SNA is used to study social influence, social cohesion, and community structure, shedding light on phenomena such as social capital, homophily, and social contagion. In organizational studies, SNA helps analyze communication patterns, collaboration networks, and knowledge sharing within organizations, informing strategies for improving organizational effectiveness and innovation. In epidemiology, SNA is applied to understand disease transmission, identify influential individuals or groups, and design targeted interventions for disease prevention and control. In information science, SNA is used to analyze online social networks, study information diffusion, and detect misinformation or disinformation campaigns. In marketing, SNA assists in identifying key opinion leaders, understanding customer behavior, and optimizing marketing strategies. In cybersecurity, SNA helps detect and mitigate security threats, analyze cyber-attack patterns, and protect critical infrastructure from malicious actors.
Challenges and Future Directions
Despite its utility, Social Network Analysis faces several challenges, including issues related to data collection, network representation, model complexity, and ethical considerations. Future research directions include advancing methods for analyzing dynamic and multimodal networks, integrating SNA with other analytical approaches such as machine learning and natural language processing, addressing algorithmic bias and fairness concerns, and developing ethical guidelines for conducting SNA research.
Conclusion
Social Network Analysis offers a powerful framework for studying social structures, interactions, and dynamics across diverse domains. By leveraging concepts and techniques from graph theory, statistics, and computational methods, SNA enables researchers to uncover hidden patterns, identify influential actors, and understand emergent phenomena in social networks. Continued research and innovation in Social Network Analysis are essential for addressing complex societal challenges and advancing our understanding of human behavior and social systems.
0
3
0
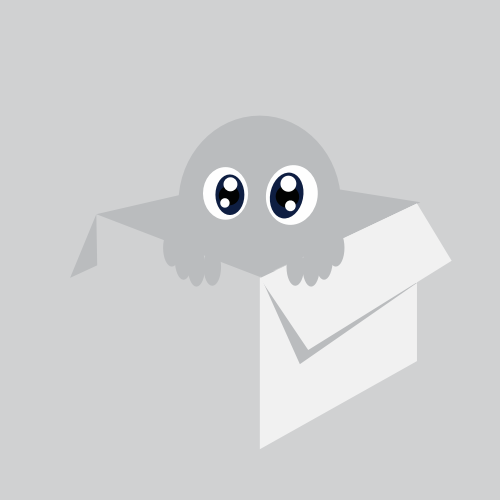
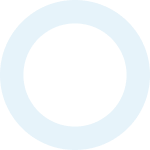
Komentar yang asik ya
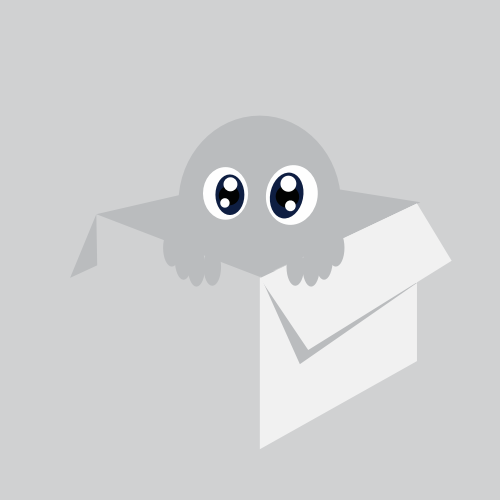
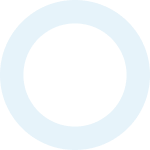
Komentar yang asik ya
Komunitas Pilihan