- Beranda
- Komunitas
- Story
- penelitian
Literature Review and Theoretical Review of Neurosymbolic AI Introduction
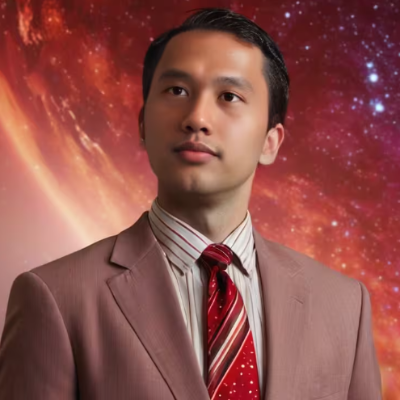
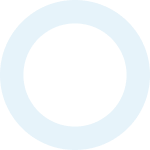
TS
yuliuseka
Literature Review and Theoretical Review of Neurosymbolic AI Introduction
[color=var(--tw-prose-body)][color=var(--tw-prose-headings)]Literature Review and Theoretical Review of Neurosymbolic AI
Introduction
Neurosymbolic AI is an interdisciplinary field that integrates symbolic reasoning with neural networks to address complex problems requiring both symbolic and subsymbolic processing. This review provides an overview of the theoretical foundations, methodologies, applications, and challenges associated with Neurosymbolic AI.
Literature Review
Historical Development
The concept of Neurosymbolic AI has roots in both symbolic AI, which emphasizes logical reasoning and knowledge representation, and connectionist AI, which focuses on neural network-based learning from data. The integration of symbolic reasoning and neural networks gained momentum in the late 20th and early 21st centuries as researchers sought to combine the strengths of both approaches. Early work in Neurosymbolic AI includes hybrid systems that incorporate symbolic rules into neural network architectures and vice versa.
Key Concepts and Techniques
Symbolic Reasoning:
Symbolic reasoning involves manipulating abstract symbols according to logical rules. It enables precise, rule-based reasoning and knowledge representation, making it suitable for tasks requiring explicit, interpretable representations of knowledge and logic-based decision-making.
Neural Networks:
Neural networks are computational models inspired by the structure and function of the brain. They consist of interconnected nodes (neurons) organized in layers, capable of learning complex patterns and relationships from data through training algorithms such as backpropagation.
Integration Strategies:
Neurosymbolic AI integrates symbolic reasoning with neural networks through various strategies, including:
Neural-Symbolic Integration: Embedding symbolic knowledge into neural network architectures or incorporating neural representations into symbolic reasoning systems.
Hybrid Models: Combining separate symbolic and neural components within a unified framework to leverage the strengths of both approaches.
Interpretable Models: Designing models that produce human-interpretable explanations of their decisions, facilitating transparency and trust.
Applications of Neurosymbolic AI
Neurosymbolic AI has diverse applications across domains such as natural language understanding, robotics, healthcare, finance, and scientific discovery. In natural language understanding, Neurosymbolic models can combine neural representations of text with symbolic reasoning to perform tasks such as semantic parsing, question answering, and text generation. In robotics, Neurosymbolic approaches enable robots to reason about high-level concepts and symbolic goals while leveraging neural networks for perception and control. In healthcare, Neurosymbolic techniques support medical diagnosis, treatment planning, and drug discovery by integrating clinical knowledge with data-driven insights from patient records and biomedical literature. In finance, Neurosymbolic models aid in risk assessment, portfolio management, and fraud detection by combining financial rules and regulations with machine learning-based prediction and analysis. In scientific discovery, Neurosymbolic methods facilitate hypothesis generation, data interpretation, and knowledge discovery by integrating domain-specific knowledge with data-driven insights from experimental and observational data.
Challenges and Future Directions
Despite its potential, Neurosymbolic AI faces challenges related to knowledge representation, learning scalability, interpretability, and integration complexity. Future research directions include developing more efficient and scalable Neurosymbolic architectures, improving the interpretability and transparency of hybrid models, addressing the semantic gap between symbolic and subsymbolic representations, and advancing techniques for integrating heterogeneous sources of knowledge and data.
Conclusion
Neurosymbolic AI represents a promising approach to AI that combines the strengths of symbolic reasoning and neural networks to tackle complex problems requiring both logical inference and statistical learning. By integrating symbolic knowledge with neural representations, Neurosymbolic AI enables more interpretable, transparent, and context-aware AI systems across various domains. Continued research and innovation in Neurosymbolic AI are essential for addressing existing challenges and unlocking new opportunities for AI-driven problem-solving and decision-making.[/color][/color]
Introduction
Neurosymbolic AI is an interdisciplinary field that integrates symbolic reasoning with neural networks to address complex problems requiring both symbolic and subsymbolic processing. This review provides an overview of the theoretical foundations, methodologies, applications, and challenges associated with Neurosymbolic AI.
Literature Review
Historical Development
The concept of Neurosymbolic AI has roots in both symbolic AI, which emphasizes logical reasoning and knowledge representation, and connectionist AI, which focuses on neural network-based learning from data. The integration of symbolic reasoning and neural networks gained momentum in the late 20th and early 21st centuries as researchers sought to combine the strengths of both approaches. Early work in Neurosymbolic AI includes hybrid systems that incorporate symbolic rules into neural network architectures and vice versa.
Key Concepts and Techniques
Symbolic Reasoning:
Symbolic reasoning involves manipulating abstract symbols according to logical rules. It enables precise, rule-based reasoning and knowledge representation, making it suitable for tasks requiring explicit, interpretable representations of knowledge and logic-based decision-making.
Neural Networks:
Neural networks are computational models inspired by the structure and function of the brain. They consist of interconnected nodes (neurons) organized in layers, capable of learning complex patterns and relationships from data through training algorithms such as backpropagation.
Integration Strategies:
Neurosymbolic AI integrates symbolic reasoning with neural networks through various strategies, including:
Neural-Symbolic Integration: Embedding symbolic knowledge into neural network architectures or incorporating neural representations into symbolic reasoning systems.
Hybrid Models: Combining separate symbolic and neural components within a unified framework to leverage the strengths of both approaches.
Interpretable Models: Designing models that produce human-interpretable explanations of their decisions, facilitating transparency and trust.
Applications of Neurosymbolic AI
Neurosymbolic AI has diverse applications across domains such as natural language understanding, robotics, healthcare, finance, and scientific discovery. In natural language understanding, Neurosymbolic models can combine neural representations of text with symbolic reasoning to perform tasks such as semantic parsing, question answering, and text generation. In robotics, Neurosymbolic approaches enable robots to reason about high-level concepts and symbolic goals while leveraging neural networks for perception and control. In healthcare, Neurosymbolic techniques support medical diagnosis, treatment planning, and drug discovery by integrating clinical knowledge with data-driven insights from patient records and biomedical literature. In finance, Neurosymbolic models aid in risk assessment, portfolio management, and fraud detection by combining financial rules and regulations with machine learning-based prediction and analysis. In scientific discovery, Neurosymbolic methods facilitate hypothesis generation, data interpretation, and knowledge discovery by integrating domain-specific knowledge with data-driven insights from experimental and observational data.
Challenges and Future Directions
Despite its potential, Neurosymbolic AI faces challenges related to knowledge representation, learning scalability, interpretability, and integration complexity. Future research directions include developing more efficient and scalable Neurosymbolic architectures, improving the interpretability and transparency of hybrid models, addressing the semantic gap between symbolic and subsymbolic representations, and advancing techniques for integrating heterogeneous sources of knowledge and data.
Conclusion
Neurosymbolic AI represents a promising approach to AI that combines the strengths of symbolic reasoning and neural networks to tackle complex problems requiring both logical inference and statistical learning. By integrating symbolic knowledge with neural representations, Neurosymbolic AI enables more interpretable, transparent, and context-aware AI systems across various domains. Continued research and innovation in Neurosymbolic AI are essential for addressing existing challenges and unlocking new opportunities for AI-driven problem-solving and decision-making.[/color][/color]
0
9
1
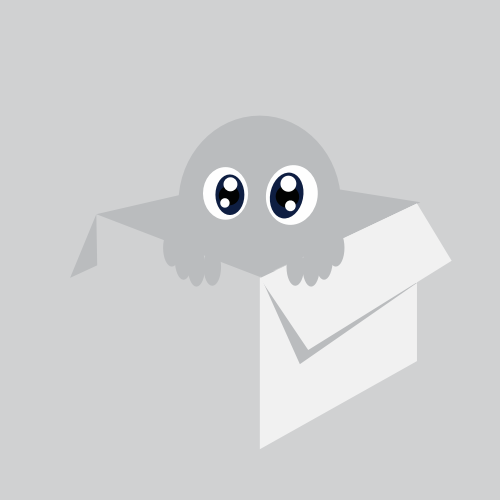
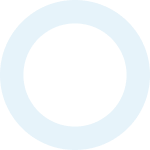
Komentar yang asik ya
Urutan
Terbaru
Terlama
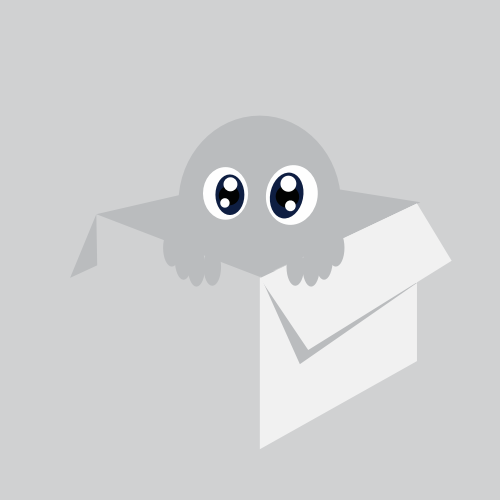
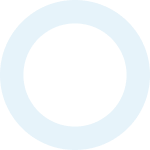
Komentar yang asik ya
Komunitas Pilihan