- Beranda
- Komunitas
- Story
- penelitian
Literature Review and Theoretical Review of Differential Privacy
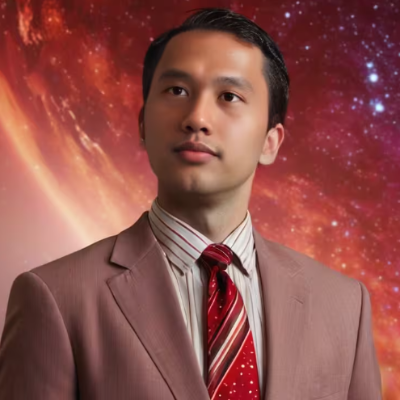
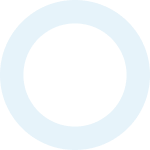
TS
yuliuseka
Literature Review and Theoretical Review of Differential Privacy
Literature Review and Theoretical Review of Differential Privacy
Introduction
Differential Privacy is a rigorous privacy framework that ensures the confidentiality of sensitive information in data analysis and machine learning tasks. This review provides an overview of the theoretical foundations, methodologies, applications, and challenges associated with Differential Privacy.
Literature Review
Historical Development
Differential Privacy emerged from the need to protect individuals' privacy while enabling valuable data analysis. Early research in Differential Privacy dates back to the mid-2000s, with seminal works by Cynthia Dwork and colleagues, who formalized the concept and established its mathematical foundations. Since then, Differential Privacy has gained significant attention from both academia and industry, leading to advancements in privacy-preserving techniques and their applications.
Key Concepts and Techniques
[color=var(--tw-prose-bold)]Privacy Guarantees:
Differential Privacy provides strong privacy guarantees by ensuring that the presence or absence of any individual's data does not significantly impact the outcome of data analysis. This is achieved by adding carefully calibrated noise to query responses or by perturbing the input data before analysis.
Noise Addition Mechanisms:
Differential Privacy mechanisms employ various noise addition techniques, such as Laplace noise for numerical data and exponential noise for categorical data. The amount of noise added is determined by the desired privacy level and the sensitivity of the query being executed.
Privacy Budget:
The concept of privacy budget quantifies the privacy loss incurred by multiple queries or computations on the same dataset. By limiting the cumulative privacy loss within a predefined budget, Differential Privacy ensures long-term privacy guarantees while allowing for useful data analysis.
[/color]
Applications of Differential Privacy
Differential Privacy finds applications in diverse domains, including healthcare, finance, social sciences, and data-driven policymaking. In healthcare, Differential Privacy protects patients' sensitive medical records while enabling researchers to perform population-level analysis and disease surveillance. In finance, Differential Privacy safeguards individuals' financial transactions and credit histories while allowing for fraud detection and risk assessment. In social sciences, Differential Privacy preserves respondents' privacy in survey data analysis while preserving the integrity of statistical analyses. In policymaking, Differential Privacy supports evidence-based decision-making by enabling the analysis of sensitive government datasets while protecting individuals' privacy.
Challenges and Future Directions
Despite its advantages, Differential Privacy faces challenges related to utility-privacy trade-offs, scalability, and usability. Future research directions include developing more efficient Differential Privacy mechanisms, addressing the challenges of data heterogeneity and high-dimensional data, and integrating Differential Privacy into standard data analysis and machine learning workflows. Additionally, efforts are needed to raise awareness and promote adoption of Differential Privacy principles among data practitioners and policymakers.
Conclusion
Differential Privacy offers a principled approach to privacy preservation in data analysis and machine learning, ensuring that sensitive information remains protected while enabling valuable insights to be extracted from data. By providing strong privacy guarantees and enabling privacy-preserving data analysis, Differential Privacy contributes to building trust and accountability in data-driven applications across various domains. Continued research and innovation in Differential Privacy are essential for addressing emerging privacy challenges and advancing the state-of-the-art in privacy-preserving techniques.
Introduction
Differential Privacy is a rigorous privacy framework that ensures the confidentiality of sensitive information in data analysis and machine learning tasks. This review provides an overview of the theoretical foundations, methodologies, applications, and challenges associated with Differential Privacy.
Literature Review
Historical Development
Differential Privacy emerged from the need to protect individuals' privacy while enabling valuable data analysis. Early research in Differential Privacy dates back to the mid-2000s, with seminal works by Cynthia Dwork and colleagues, who formalized the concept and established its mathematical foundations. Since then, Differential Privacy has gained significant attention from both academia and industry, leading to advancements in privacy-preserving techniques and their applications.
Key Concepts and Techniques
[color=var(--tw-prose-bold)]Privacy Guarantees:
Differential Privacy provides strong privacy guarantees by ensuring that the presence or absence of any individual's data does not significantly impact the outcome of data analysis. This is achieved by adding carefully calibrated noise to query responses or by perturbing the input data before analysis.
Noise Addition Mechanisms:
Differential Privacy mechanisms employ various noise addition techniques, such as Laplace noise for numerical data and exponential noise for categorical data. The amount of noise added is determined by the desired privacy level and the sensitivity of the query being executed.
Privacy Budget:
The concept of privacy budget quantifies the privacy loss incurred by multiple queries or computations on the same dataset. By limiting the cumulative privacy loss within a predefined budget, Differential Privacy ensures long-term privacy guarantees while allowing for useful data analysis.
[/color]
Applications of Differential Privacy
Differential Privacy finds applications in diverse domains, including healthcare, finance, social sciences, and data-driven policymaking. In healthcare, Differential Privacy protects patients' sensitive medical records while enabling researchers to perform population-level analysis and disease surveillance. In finance, Differential Privacy safeguards individuals' financial transactions and credit histories while allowing for fraud detection and risk assessment. In social sciences, Differential Privacy preserves respondents' privacy in survey data analysis while preserving the integrity of statistical analyses. In policymaking, Differential Privacy supports evidence-based decision-making by enabling the analysis of sensitive government datasets while protecting individuals' privacy.
Challenges and Future Directions
Despite its advantages, Differential Privacy faces challenges related to utility-privacy trade-offs, scalability, and usability. Future research directions include developing more efficient Differential Privacy mechanisms, addressing the challenges of data heterogeneity and high-dimensional data, and integrating Differential Privacy into standard data analysis and machine learning workflows. Additionally, efforts are needed to raise awareness and promote adoption of Differential Privacy principles among data practitioners and policymakers.
Conclusion
Differential Privacy offers a principled approach to privacy preservation in data analysis and machine learning, ensuring that sensitive information remains protected while enabling valuable insights to be extracted from data. By providing strong privacy guarantees and enabling privacy-preserving data analysis, Differential Privacy contributes to building trust and accountability in data-driven applications across various domains. Continued research and innovation in Differential Privacy are essential for addressing emerging privacy challenges and advancing the state-of-the-art in privacy-preserving techniques.
0
4
1
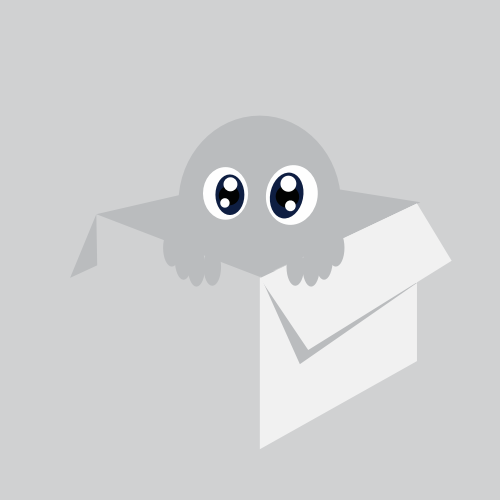
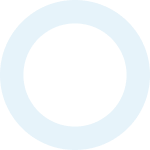
Komentar yang asik ya
Urutan
Terbaru
Terlama
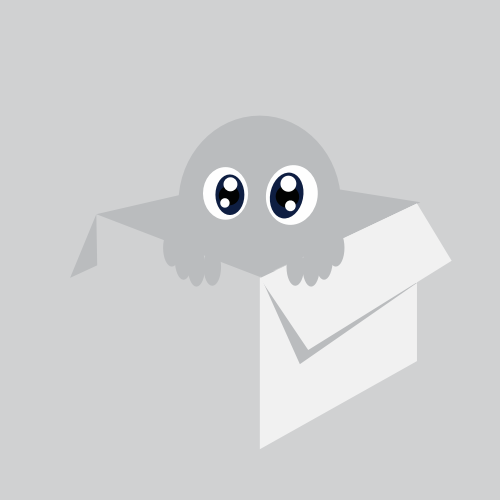
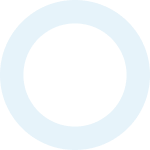
Komentar yang asik ya
Komunitas Pilihan