- Beranda
- Komunitas
- Story
- penelitian
Literature Review and Theoretical Review of Explainable Reinforcement Learning (XRL)
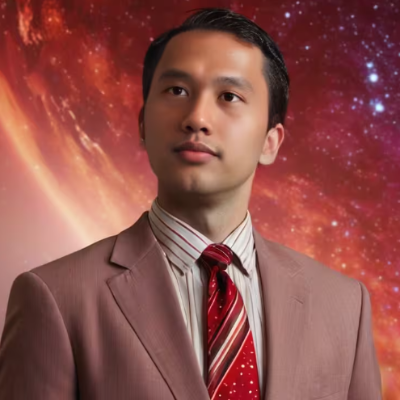
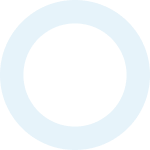
TS
yuliuseka
Literature Review and Theoretical Review of Explainable Reinforcement Learning (XRL)
Literature Review and Theoretical Review of Explainable Reinforcement Learning (XRL)
Introduction
Explainable Reinforcement Learning (XRL) is a specialized field within reinforcement learning (RL) that aims to enhance the transparency and interpretability of RL models and their decision-making processes. This review provides an overview of the theoretical foundations, methodologies, applications, and challenges associated with Explainable Reinforcement Learning.
Literature Review
Historical Development
Explainable Reinforcement Learning has gained significance due to the growing adoption of RL in real-world applications where interpretability and transparency are critical. Early research primarily focused on post-hoc interpretation techniques, such as feature attribution and saliency maps, to understand RL agent behavior. Recent advancements integrate explainability directly into RL algorithms, enabling agents to provide real-time explanations of their actions and policies.
Key Concepts and Techniques
[color=var(--tw-prose-bold)]Interpretable Models:
XRL employs interpretable models like decision trees, linear models, or rule-based systems to represent learned policies or value functions. These models facilitate human understanding of the agent's decision-making process, ensuring transparency and accountability.
Local and Global Explanations:
XRL provides both local and global explanations, allowing stakeholders to understand individual action decisions and the overall policy strategy. Local explanations focus on justifying specific agent actions, while global explanations provide insights into the agent's overall behavior.
Attention Mechanisms:
Attention mechanisms in XRL models highlight relevant features or states that influence the agent's decision-making process. By visualizing attention weights, stakeholders can identify critical factors driving the agent's behavior.
[/color]
Applications of Explainable Reinforcement Learning
XRL finds applications in various domains, including robotics, healthcare, finance, and autonomous systems. In robotics, XRL enables human-robot collaboration by providing interpretable explanations of robot actions. In healthcare, XRL models help clinicians understand AI-driven treatment recommendations and diagnosis decisions. In finance, XRL enhances algorithmic trading systems by providing transparent insights into trading strategies. In autonomous systems, XRL ensures safety and reliability by allowing humans to understand and trust AI-driven decisions.
Challenges and Future Directions
Despite its potential, XRL faces challenges related to scalability, performance, and model complexity. Future research directions include developing more scalable XRL algorithms, improving the interpretability of deep RL models, and addressing ethical considerations surrounding XRL deployment in sensitive domains.
Introduction
Explainable Reinforcement Learning (XRL) is a specialized field within reinforcement learning (RL) that aims to enhance the transparency and interpretability of RL models and their decision-making processes. This review provides an overview of the theoretical foundations, methodologies, applications, and challenges associated with Explainable Reinforcement Learning.
Literature Review
Historical Development
Explainable Reinforcement Learning has gained significance due to the growing adoption of RL in real-world applications where interpretability and transparency are critical. Early research primarily focused on post-hoc interpretation techniques, such as feature attribution and saliency maps, to understand RL agent behavior. Recent advancements integrate explainability directly into RL algorithms, enabling agents to provide real-time explanations of their actions and policies.
Key Concepts and Techniques
[color=var(--tw-prose-bold)]Interpretable Models:
XRL employs interpretable models like decision trees, linear models, or rule-based systems to represent learned policies or value functions. These models facilitate human understanding of the agent's decision-making process, ensuring transparency and accountability.
Local and Global Explanations:
XRL provides both local and global explanations, allowing stakeholders to understand individual action decisions and the overall policy strategy. Local explanations focus on justifying specific agent actions, while global explanations provide insights into the agent's overall behavior.
Attention Mechanisms:
Attention mechanisms in XRL models highlight relevant features or states that influence the agent's decision-making process. By visualizing attention weights, stakeholders can identify critical factors driving the agent's behavior.
[/color]
Applications of Explainable Reinforcement Learning
XRL finds applications in various domains, including robotics, healthcare, finance, and autonomous systems. In robotics, XRL enables human-robot collaboration by providing interpretable explanations of robot actions. In healthcare, XRL models help clinicians understand AI-driven treatment recommendations and diagnosis decisions. In finance, XRL enhances algorithmic trading systems by providing transparent insights into trading strategies. In autonomous systems, XRL ensures safety and reliability by allowing humans to understand and trust AI-driven decisions.
Challenges and Future Directions
Despite its potential, XRL faces challenges related to scalability, performance, and model complexity. Future research directions include developing more scalable XRL algorithms, improving the interpretability of deep RL models, and addressing ethical considerations surrounding XRL deployment in sensitive domains.
0
5
2
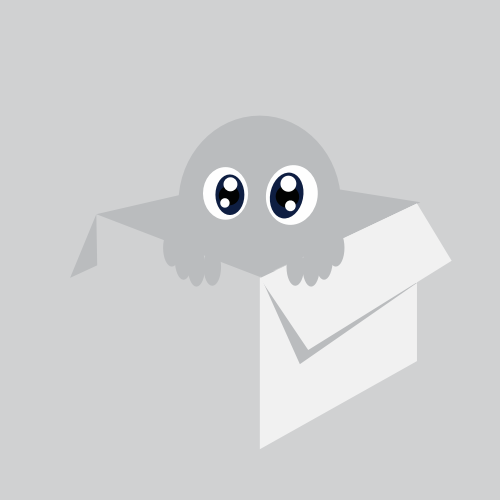
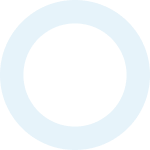
Komentar yang asik ya
Urutan
Terbaru
Terlama
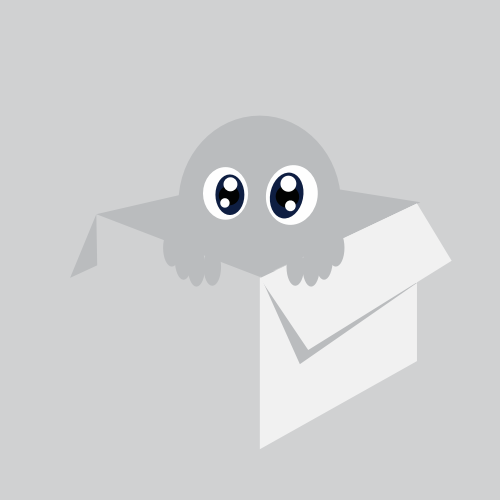
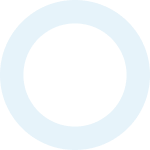
Komentar yang asik ya
Komunitas Pilihan