- Beranda
- Komunitas
- Story
- penelitian
Literature Review and Theoretical Review of Quantum Machine Learning
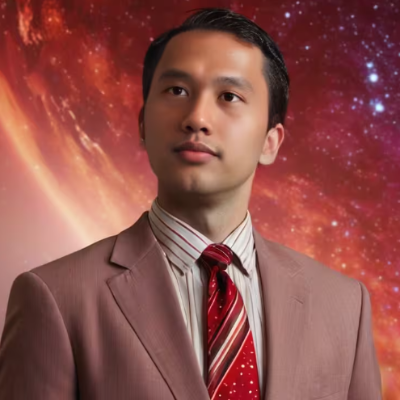
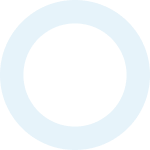
TS
yuliuseka
Literature Review and Theoretical Review of Quantum Machine Learning
Literature Review and Theoretical Review of Quantum Machine Learning
Introduction
Quantum machine learning is an interdisciplinary field that explores the intersection of quantum computing and machine learning. This review provides an overview of the theoretical foundations, methodologies, applications, and challenges associated with quantum machine learning.
Literature Review
Historical Development
Quantum machine learning emerged from the convergence of quantum computing, quantum information theory, and classical machine learning. Early work in quantum machine learning dates back to the late 1990s, with researchers exploring the potential of quantum algorithms, such as quantum neural networks and quantum support vector machines, to outperform classical machine learning approaches on certain tasks.
Key Concepts and Techniques
[color=var(--tw-prose-bold)]Quantum Computing Basics:
Quantum machine learning relies on principles from quantum mechanics, such as superposition, entanglement, and interference, to perform computations on quantum data. Quantum computers leverage quantum bits (qubits) as fundamental units of information, enabling parallel processing and exponential speedup for certain algorithms.
Quantum-enhanced Algorithms:
Quantum machine learning algorithms aim to harness the computational power of quantum computers to solve optimization, sampling, and pattern recognition problems more efficiently than classical algorithms. Techniques such as quantum annealing, quantum walk, and variational quantum circuits are used to design quantum-enhanced machine learning algorithms.
Quantum Data Representation:
Quantum machine learning requires novel approaches to represent and process quantum data, which often exhibit complex quantum correlations and entanglement structures. Quantum feature maps, quantum kernels, and quantum embeddings enable the encoding of classical data into quantum states suitable for processing on quantum computers.
[/color]
Applications of Quantum Machine Learning
Quantum machine learning has applications in various fields, including optimization, cryptography, drug discovery, and material science. In optimization, quantum algorithms offer the potential for exponential speedup compared to classical algorithms, enabling the solution of large-scale optimization problems more efficiently. In cryptography, quantum machine learning techniques contribute to the development of quantum-safe encryption methods and secure communication protocols resistant to quantum attacks. In drug discovery, quantum machine learning facilitates the simulation of molecular structures and the prediction of chemical properties, accelerating the drug development process. In material science, quantum machine learning enables the discovery of novel materials with desired properties through quantum simulation and optimization techniques.
Challenges and Future Directions
Quantum machine learning faces several challenges, including qubit decoherence, gate errors, limited qubit connectivity, and the requirement for error correction. Future research directions in quantum machine learning include improving qubit coherence and gate fidelity, developing fault-tolerant quantum computing architectures, exploring quantum algorithms for specific machine learning tasks, and integrating quantum machine learning with classical computing methods for hybrid quantum-classical algorithms.
Introduction
Quantum machine learning is an interdisciplinary field that explores the intersection of quantum computing and machine learning. This review provides an overview of the theoretical foundations, methodologies, applications, and challenges associated with quantum machine learning.
Literature Review
Historical Development
Quantum machine learning emerged from the convergence of quantum computing, quantum information theory, and classical machine learning. Early work in quantum machine learning dates back to the late 1990s, with researchers exploring the potential of quantum algorithms, such as quantum neural networks and quantum support vector machines, to outperform classical machine learning approaches on certain tasks.
Key Concepts and Techniques
[color=var(--tw-prose-bold)]Quantum Computing Basics:
Quantum machine learning relies on principles from quantum mechanics, such as superposition, entanglement, and interference, to perform computations on quantum data. Quantum computers leverage quantum bits (qubits) as fundamental units of information, enabling parallel processing and exponential speedup for certain algorithms.
Quantum-enhanced Algorithms:
Quantum machine learning algorithms aim to harness the computational power of quantum computers to solve optimization, sampling, and pattern recognition problems more efficiently than classical algorithms. Techniques such as quantum annealing, quantum walk, and variational quantum circuits are used to design quantum-enhanced machine learning algorithms.
Quantum Data Representation:
Quantum machine learning requires novel approaches to represent and process quantum data, which often exhibit complex quantum correlations and entanglement structures. Quantum feature maps, quantum kernels, and quantum embeddings enable the encoding of classical data into quantum states suitable for processing on quantum computers.
[/color]
Applications of Quantum Machine Learning
Quantum machine learning has applications in various fields, including optimization, cryptography, drug discovery, and material science. In optimization, quantum algorithms offer the potential for exponential speedup compared to classical algorithms, enabling the solution of large-scale optimization problems more efficiently. In cryptography, quantum machine learning techniques contribute to the development of quantum-safe encryption methods and secure communication protocols resistant to quantum attacks. In drug discovery, quantum machine learning facilitates the simulation of molecular structures and the prediction of chemical properties, accelerating the drug development process. In material science, quantum machine learning enables the discovery of novel materials with desired properties through quantum simulation and optimization techniques.
Challenges and Future Directions
Quantum machine learning faces several challenges, including qubit decoherence, gate errors, limited qubit connectivity, and the requirement for error correction. Future research directions in quantum machine learning include improving qubit coherence and gate fidelity, developing fault-tolerant quantum computing architectures, exploring quantum algorithms for specific machine learning tasks, and integrating quantum machine learning with classical computing methods for hybrid quantum-classical algorithms.
0
6
1
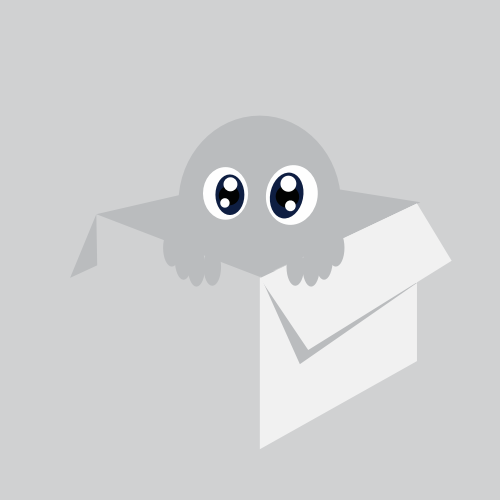
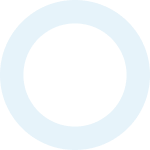
Komentar yang asik ya
Urutan
Terbaru
Terlama
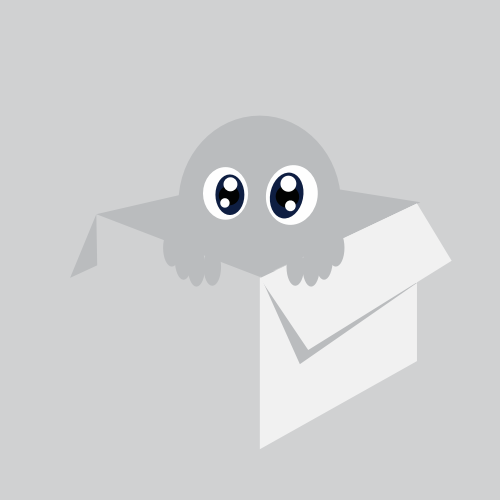
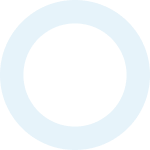
Komentar yang asik ya
Komunitas Pilihan