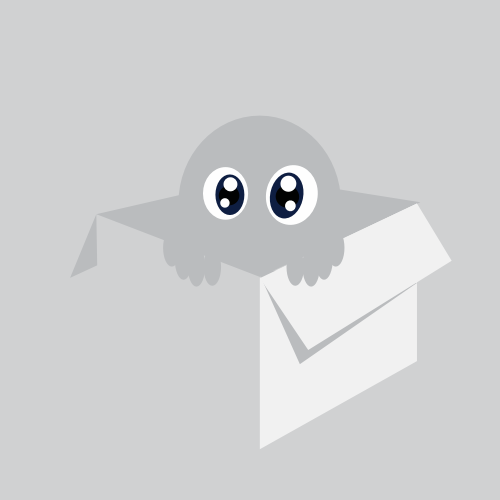
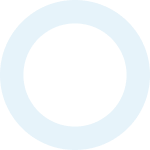
TS
swethakrishn017
Introducing Predictive Modeling for Data Science Tasks
Introduction
Predictive modeling is a critical part of data science. It is necessary to gain a thorough understanding of the data and make decisions that will propel the business. One of the last phases of data science requires you to produce predictions based on past data.
Statistics are used in predictive modeling to project the results. As a result, both data science and predictive modeling have a statistical foundation. Predictive modeling is one of the many data operations that make up data science. Machine learning and predictive modeling largely have similar boundaries. Finding patterns and predicting results are thus two essential capabilities of predictive modeling.
Types of Predictive Models
There are two types of predictive modeling.
1. Predictive Parametric Modeling
2. Non-Predictive Parametric Modeling
Predictive Parametric Modeling:
The model used in parametric predictive modeling is finite-dimensional and has a fixed size. The quantity of training examples has no bearing on a parametric predictive model. Therefore, the model's requirements for the parameters will not change regardless of how much data is assigned to it.
Non-Predictive Parametric Modeling:
Models of this kind don't rely on any parametric boundaries. They do not make any firm assumptions regarding the structure of mapping functions. They can freely learn any functionality from the training data because they don't make any assumptions. They function best when you have a lot of data but lack knowledge. Non-parametric models learn the functional forms from training data in such circumstances.
Visit the data science course for more in-depth insights on the various classifications of predictive modeling.
Data Science Procedure for Creating Predictive Model
1. Creation of a Predictive Model- Using a variety of software programs and tools, you can build a predictive model that will be used to run algorithms on a dataset.
2. Testing the model- We test the model on historical data to determine how well it performs.
3. Model Validation- To run the model using visualization tools for better comprehension, we must be able to validate it.
4. Model Evaluation- Ultimately, we assess the best fit model and decide that it is the best option for solving the issue.
The Future of Predictive modeling
Three major trends will influence the future of data modeling.
1. More business applications and citizen data science tools now include data modeling functionality. These capabilities can give business users the proper guidelines and templates to use when working with predictive modeling.
2. Low-code predictive modeling tools and frameworks make it simpler for data science experts to quickly clean up data, build models, and validate the outcomes.
3. More advanced tools are being developed to automate many of the data engineering tasks necessary to put predictive models into use. According to Carroll, more organizations will be able to deploy their models in ways that maximize their potential value.
Summary
By looking for patterns in a set of input data, predictive modeling uses mathematics to foretell future events or results. It is an essential part of predictive analytics—a type of data science and data analytics that uses both recent and old data to predict activity, behavior, and trends.
Are you thinking about becoming a data scientist? The platform you need might be the Learnbay training institute. Learnbay, which provides industry-accredited data science course in Pune and is co-powered by IBM, involves students in real-world projects created by professionals in the industry.
0
222
0
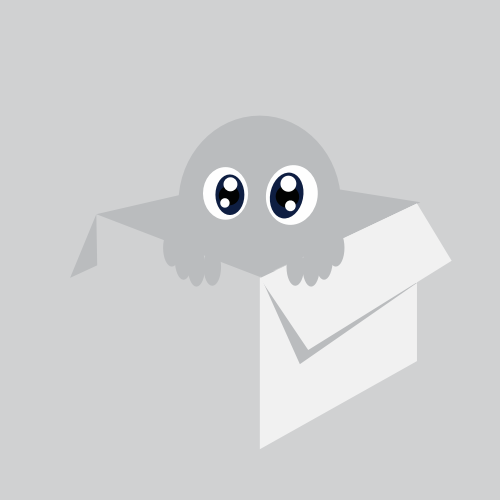
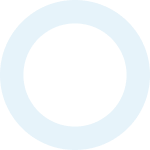
Komentar yang asik ya
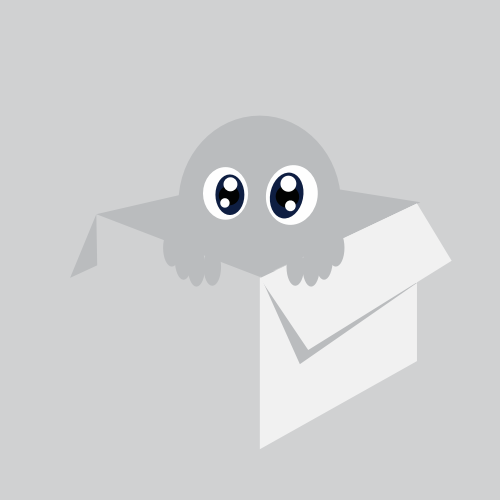
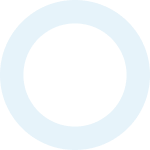
Komentar yang asik ya
Komunitas Pilihan