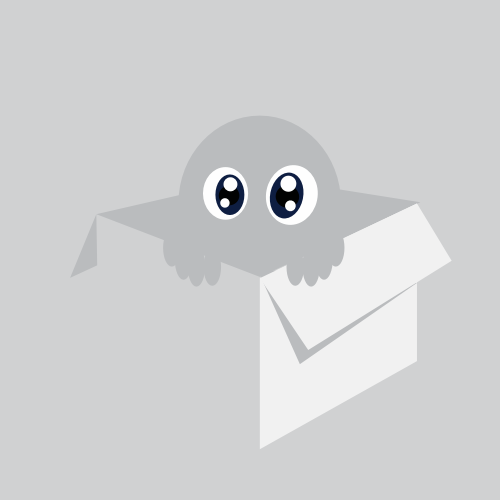
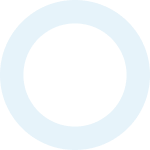
TS
ashsteinfeld245
The Role of Data Science in Fintech
A whole new paradigm has emerged as a result of the real-world application of statistics and computation. As a result, the field of study known as data science is in the process of making every activity, from business to media, more effective and fruitful.
The financial sector is the only one where data science has successfully brought about change. Fintech is a new financial animal species that data science has produced. By enabling the use of massive data and intricate computations in financial decision-making, fintech has drastically changed the financial environment. Moreover, the application of data science to financial decision-making has significantly increased accuracy and predictive power across the board.
What is Data Science?
Large-scale organized and unstructured data analysis using cutting-edge analytical methods is known as data science. The amount of data gathered in 2021 increased by 37% over 2020, totaling 74 trillion gigabytes. The statistics are quite stunning.
The volume of data on the Internet
The variety of data being collected has increased along with the volume of data. This makes it challenging to analyze and organize information, which would be difficult for humans. Data science can help with that.
Companies can employ data science to get a thorough data analysis, projections, and insights that can be applied to every aspect of the organization's processes.
Data science combines various disciplines, including:
statistics;
mathematics;
quantitative finance;
data analysis;
algorithmic development; and
Visualization.
Use Cases of Data Science in Fintech
Some of the most popular use cases for data science in financial technology are discussed below.
Fraud Prevention
Every financial business fears the worst—fraudsters taking both money and company information. They are therefore defending information with every anti-fraud weapon at their disposal.
For instance, businesses can use regulatory technology, or Regtech, to improve security measures. This comprises:
Blockchain technologies enhance the security of direct transactions, intelligence applications for anti-money laundering (AML), a single-risk platform for end-to-end fraud protection, and machine learning for communication monitoring and client identification.
Risk Analysis
Deep risk analysis is provided by data science to a business, and by incorporating new risk detection system features, data science aids in risk management.
By replicating certain hazards in a virtual environment, businesses can get ready for future issues. They could use risk analysis, for instance, to determine whether a potential borrower has a good chance of paying back the loan. In addition, companies can better understand the risk prerequisites and manage them across the organization by using risk simulation.
Based on the past behavior of consumer transactions, data science develops a risk model. It aids in determining the dependability of consumers and, if so, allows for the provision of advantages like discounted prices and more services.
Customer Behavior Analysis
Text analysis, NLP, and data mining are used in data science to analyze a substantial amount of behavioral data.
Financial companies can increase cross-selling by providing customers with additional products or services they might find interesting, estimating and raising client lifetime value, cutting losses by reducing below-zero-value customers, segmenting customers, and improving the company's reputation as a reliable partner.
Data science offers the chance to integrate real-time and predictive analytics with the ability to model customer behavior.
Examples of Fintech Data-Driven Projects
Here are a few instances of businesses that successfully incorporated financial Data Science into their daily operations.
DBS and personalized recommendations
DBS bank has recently made significant investments in data-driven and artificial intelligence technology. As a result, the bank offers its clients individualized tips for handling their finances based on personalized insights into their behavior. In addition, the bank can identify anomalous transactions and raise security with the aid of these technologies.
Curve and protection from fraud risks
Another example is the Curve firm, which used a Sift Science Machine Learning solution to strengthen fraud defenses for its software that combines multiple credit cards into one. To swiftly identify and stop fraudulent activity, the platform monitors user behavior to reveal suspicious activities and signals.
Scotiabank and receipt management
Another intriguing example is using machine learning for banking data analytics, namely receipt management. Sensibill's platform, which enables users to take pictures of their invoices, has been integrated by Scotiabank for mobile banking services. Purchase documentation from clients is stored, organized, and accessible through the data science application. Users now have a practical way to manage physical and digital receipts.
Want to work on various data science projects in the finance domain? Enroll in Leanbay, which provides the best data science course in Mumbai. Learn and improve your skills by working on multiple projects and enhancing your portfolio.
Happy learning!
0
395
0
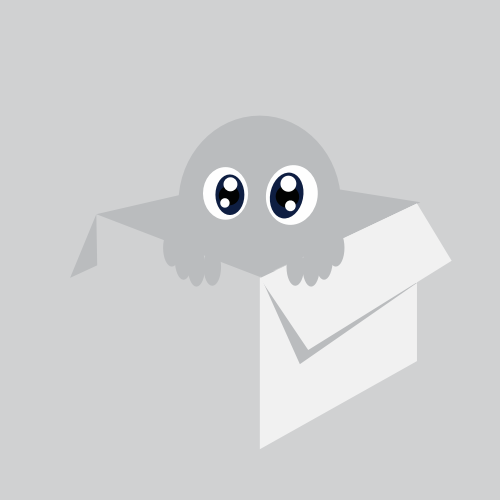
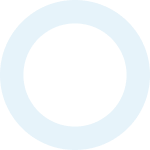
Guest
Tulis komentar menarik atau mention replykgpt untuk ngobrol seru
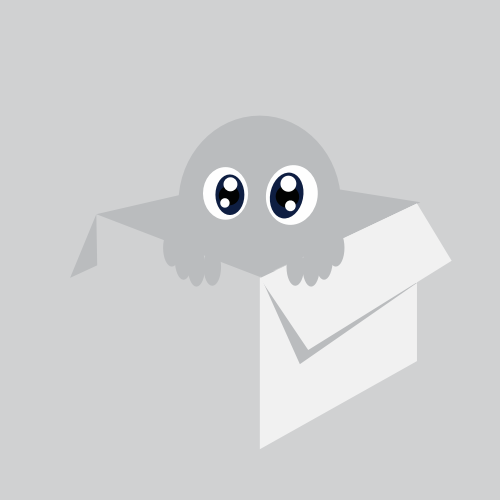
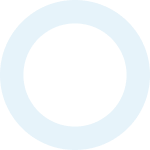
Guest
Tulis komentar menarik atau mention replykgpt untuk ngobrol seru
Komunitas Pilihan