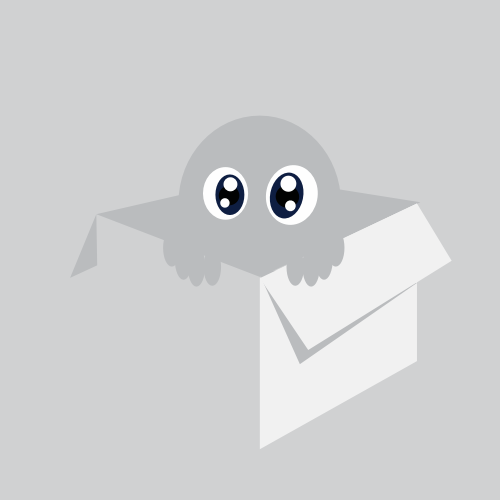
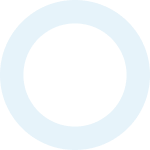
TS
daanishs078571
Is Mathematics Required To Learn Data Science?
Tired of searching sites and resources to know about the mathematics necessary to learn Data Science and AI?
Well, here we will cover the mathematics needed to learn data science. Of course, when you search for mathematics for data science and analysis, you will always come up with Calculus, Linear algebra, and statistics. However, if you want to be an immediate Data scientist who wants to be familiar with some data science job roles, you merely need Statistics and Probability.
Calculus :
Calculus also called analysis, which is the study of the rate of change of quantities in mathematics like area, length, and the volume of Objects. It is Divided into Integral and Differential.
Calculus is mostly used for Machine Learning. However, you can’t skip Calculus if you are learning Data Science.
Integral Calculus: It combines small pieces together to find how many pieces are there.
Differential Calculus: It divides something into small pieces and finds how they change.
There are many other methods like Vector-Value Functions, Directional Gradients, Partial Derivatives, and Multivariate Calculus. However, derivatives, Divergence, Curvature, and Quadratic approximations are the most important concepts you must know.
Linear algebra:
Linear algebra is also a mathematical language used in Data Science and Machine Learning. Most of the Machine learning models are expressed in matrix form.
Even sometimes, dataset lists will be represented in Matrix form. Linear algebra is used in Data processing, Model evaluation, and Data evaluation.
There are concepts you need to know,
Transpose of a matrix
The inverse of a matrix
Determinant of a matrix
Trace of a matrix
Dot product
Eigenvalues
Eigenvectors
Vectors
Matrices
Statistics and Probability:
Data science is built on the principles of probability and statistics. Making a prediction is greatly aided by the probability theory. Therefore, predictions and estimates are crucial components of data science. We generate estimates for the upcoming analysis using statistical techniques. As a result, probability theory plays a significant role in statistical procedures. Additionally, data is the basis for all probability and statistics.
Descriptive statistics:
A descriptive statistic is a summary statistic that quantifies the characteristics of a group of data or summaries them. It aids in our better understanding of our data. It is employed to explain the properties of data.
Measurement level of data:
Qualitative data - Nominal, Ordinal.
Quantitative data - Ordinal, Interval, Ratio.
Nominal: Nominal data is categorized based on Name, labels, or Quality.
Ordinal: At this level, the data will be arranged or ranked.
Interval: Data at this level can calculate the meaningful differences between the data points.
Ratio: With the additional feature of an inherent zero, data at this level are identical to the interval level. These data points can be used in mathematical calculations.
Population or Sample Data:
Before performing any analysis, we should know that the data we deal with is a population or sample.
Population: A grouping of all items (N), which comprises every single unit in our study. It is challenging to define a parameter is a measure of a characteristic like mean or mode.
Sample: A small subset of the population (n), consisting of only a few individuals. It is chosen randomly, and statistics is the measurement of the feature.
Interested in knowing more about Data Science and becoming a high-paid Data scientist? Learn the in-demand skills with the leading Data Science course in Dubai and become IBM certified data science professional.
Well, here we will cover the mathematics needed to learn data science. Of course, when you search for mathematics for data science and analysis, you will always come up with Calculus, Linear algebra, and statistics. However, if you want to be an immediate Data scientist who wants to be familiar with some data science job roles, you merely need Statistics and Probability.
Calculus :
Calculus also called analysis, which is the study of the rate of change of quantities in mathematics like area, length, and the volume of Objects. It is Divided into Integral and Differential.
Calculus is mostly used for Machine Learning. However, you can’t skip Calculus if you are learning Data Science.
Integral Calculus: It combines small pieces together to find how many pieces are there.
Differential Calculus: It divides something into small pieces and finds how they change.
There are many other methods like Vector-Value Functions, Directional Gradients, Partial Derivatives, and Multivariate Calculus. However, derivatives, Divergence, Curvature, and Quadratic approximations are the most important concepts you must know.
Linear algebra:
Linear algebra is also a mathematical language used in Data Science and Machine Learning. Most of the Machine learning models are expressed in matrix form.
Even sometimes, dataset lists will be represented in Matrix form. Linear algebra is used in Data processing, Model evaluation, and Data evaluation.
There are concepts you need to know,
Transpose of a matrix
The inverse of a matrix
Determinant of a matrix
Trace of a matrix
Dot product
Eigenvalues
Eigenvectors
Vectors
Matrices
Statistics and Probability:
Data science is built on the principles of probability and statistics. Making a prediction is greatly aided by the probability theory. Therefore, predictions and estimates are crucial components of data science. We generate estimates for the upcoming analysis using statistical techniques. As a result, probability theory plays a significant role in statistical procedures. Additionally, data is the basis for all probability and statistics.
Descriptive statistics:
A descriptive statistic is a summary statistic that quantifies the characteristics of a group of data or summaries them. It aids in our better understanding of our data. It is employed to explain the properties of data.
Measurement level of data:
Qualitative data - Nominal, Ordinal.
Quantitative data - Ordinal, Interval, Ratio.
Nominal: Nominal data is categorized based on Name, labels, or Quality.
Ordinal: At this level, the data will be arranged or ranked.
Interval: Data at this level can calculate the meaningful differences between the data points.
Ratio: With the additional feature of an inherent zero, data at this level are identical to the interval level. These data points can be used in mathematical calculations.
Population or Sample Data:
Before performing any analysis, we should know that the data we deal with is a population or sample.
Population: A grouping of all items (N), which comprises every single unit in our study. It is challenging to define a parameter is a measure of a characteristic like mean or mode.
Sample: A small subset of the population (n), consisting of only a few individuals. It is chosen randomly, and statistics is the measurement of the feature.
Interested in knowing more about Data Science and becoming a high-paid Data scientist? Learn the in-demand skills with the leading Data Science course in Dubai and become IBM certified data science professional.
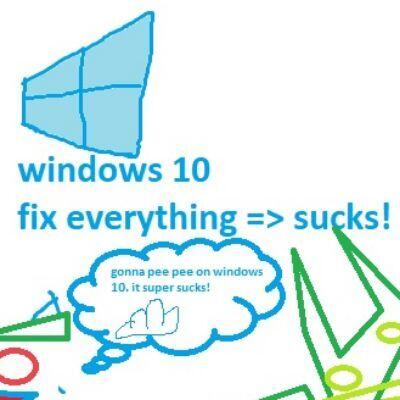
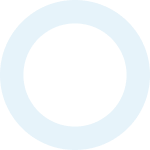
Benyamin90 memberi reputasi
1
186
1
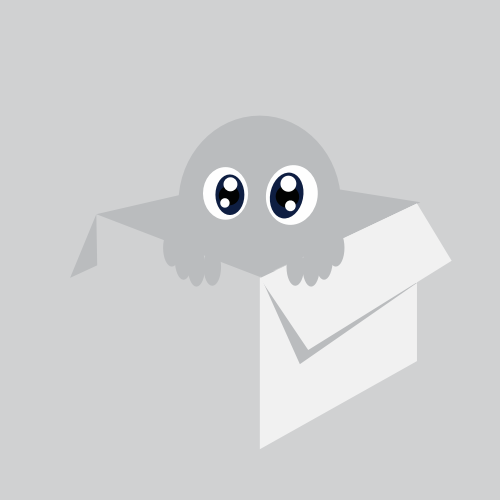
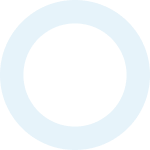
Komentar yang asik ya
Urutan
Terbaru
Terlama
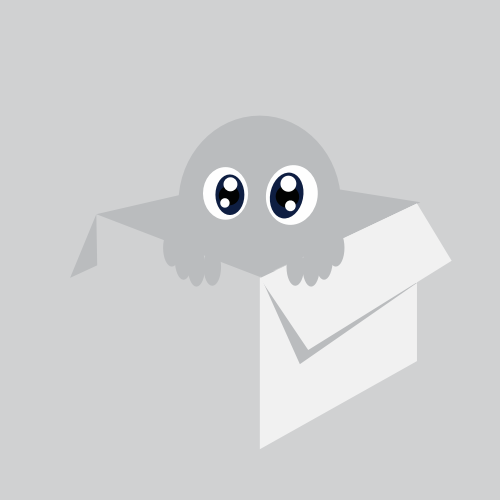
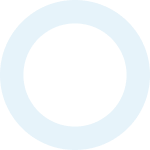
Komentar yang asik ya
Komunitas Pilihan